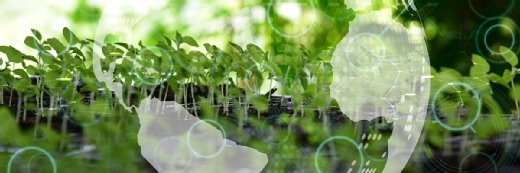
Swedish CIO contributes best practices for ethical use of artificial intelligence
IT leaders are scrambling to keep up with AI technology, but many are losing sight of its ethical impact – and what CIOs need to do to ensure responsible use
OpenAI writes on its website: “The amount of compute used in the largest AI [artificial intelligence] training runs has been increasing exponentially with a 3.4-month doubling time (by comparison, Moore’s Law had a two-year doubling period).”
According to OpenAI, this growth amounts to 300,000-times more computing power required today as opposed to 2012, which is a far greater increase than the seven-times growth of computing power you get over the same period if you assume the two-year doubling period predicted by Moore’s Law.
When you extrapolate, two things become apparent. The first is that computers will never keep up with the rise in demand from AI applications, unless some disruptive change occurs and is made available for industry. While quantum computing and in-memory computing are two potentially game-changing technologies, both are still a few years off.
The second thing that’s apparent is that, in their struggle to keep up with the demands of AI, computer manufacturers will churn out new hardware and datacentres and run everything they have at maximum power. As a result, e-waste and carbon footprints will soon get out of hand. This is the scenario we’re now in – and we’ll be here for several years to come.
According to Niklas Sundberg, chief digital officer and senior vice-president of Kuehne+Nagel, AI already has a big carbon footprint and it’s getting bigger, fast. It requires a lot of new hardware, which not only requires metals that become e-waste, but also produces a lot of carbon emissions in the manufacturing process. Moreover, running the hardware at the intensities required for both AI training and inference produces still more carbon emissions – on an ongoing basis.
Sundberg published his book, Sustainable IT playbook for technology leaders, in October 2022, about a month before OpenAI launched ChatGPT. The names of the technologies have changed, but the principles of his book still apply to the post-generative AI (GenAI) world.
On the one hand, IT leaders need to keep up with technology to ensure their organisations remain competitive. On the other, they have to act responsibly with regards to climate change – not only is it the right thing to do, but it’s also the only way they can comply with new regulation, including scope 3 reporting, which covers emissions across the supply chain.
Three ways of reducing your carbon emissions
In a recent article, Tackling AI’s climate change problem, published in the winter 2024 issue of the MIT Sloan management review, Sundberg says three best practices can be applied in the short term to minimise carbon emissions. These are what he calls the 3Rs: relocate, right-size and re-architect
Relocate datacentres to places such as Quebec, where energy is almost 100% renewable, and the average carbon intensity is 32 grams per kilowatt-hour, he writes. For American IT leaders, where the average carbon emissions for a datacentre is 519 grams per kilowatt-hour, this would result in a 16-fold reduction.
By moving on-premise IT assets to a well-architected cloud-based datacentre, organisations could save on emissions and energy by a factor of 1.4 to 2, according to Sundberg. Cloud-based datacentres are built for energy efficiency.
Right-size your AI models and applications to fit what you really need. Sundberg writes that companies can reduce their carbon footprints by right-sizing their AI models and applications, and using good archiving procedures.
If you use processors and systems designed for machine learning, instead of general-purpose hardware and platforms, you can increase both performance and efficiency by a factor of between two and five, writes Sundberg. Look for the right balance of scope, model size, model quality and efficiency. If you can schedule training periods to run at times of the day when carbon intensity tends to be lower, that also helps.
Choosing the right machine learning model architecture can also make a big difference. A sparse model, for example, can not only improve machine learning quality, but it can also decrease computing by a factor of three to 10 times.
Why cross-organisational governance is needed for AI
“When it comes to AI in general, there’s a bit of a breach of personal and societal trust,” says Sundberg. “We’re seeing implications of that with GenAI emerging quite quickly. IT has a role to play to govern that data and to make sure that it’s trustworthy. This is extremely important, and this is something that was talked about at Davos.”
According to Sundberg, while there were only 60 IT leaders among the 3,000 executives attending the event, GenAI dominated the discussion. “Everybody was talking about governance and responsible usage of AI,” he says.
Because of the broad applicability of AI, governance needs to be multidisciplinary, because of its broad applicability across business units and functional units and the outside world. It needs to be the bridge that connects organisational silos and unite different functions and leaders.
“The technology is developing very, very rapidly, and it’s really important to make sure you put an AI governance framework in place along these use cases that you’re executing or that are emerging,” says Sundberg. “CIOs [chief information officers] need to set up things such as acceptable usage policies, design principles and so forth.”
Read more about sustainability
- What started as a whisper a decade ago has become distinctly audible chatter among IT leaders sharing best practices on how to protect the environment.
- As cloud computing and digital reliance grow, so too does the need to focus on sustainability. Here’s a look at some of the issues.
- Sustainability is on many tech leaders' minds, but knowing where to start can be daunting. CIO Niklas Sundberg shares his experience building an IT sustainability plan.