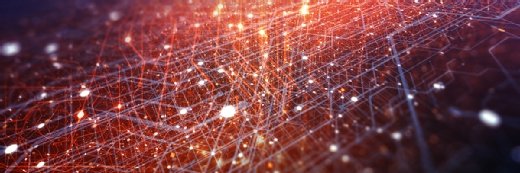
Quardia Inc. - stock.adobe.com
How generative AI and cloud complement each other
McKinsey partner explains the symbiotic relationship between generative AI and cloud, enabling organisations to speed up cloud migration and harness the benefits of AI
Generative AI (GenAI) and cloud computing are complementary capabilities that can be leveraged together to drive adoption of both technologies, according to McKinsey.
Speaking at Cloud Expo Asia in Singapore this week, Bhargs Srivathsan, a McKinsey partner and co-lead of the management consultancy’s cloud operations and optimisation work, said “cloud is needed to bring generative AI to life,” and GenAI can, in turn, simplify the migration to public cloud.
For instance, she noted that GenAI capabilities will not only help enterprises decipher and translate legacy code – such as those written in Cobol – into cloud-native languages, but also assist in modernising legacy databases in their cloud migration efforts.
“You could potentially extract the database schema or upload the DDL [data definition language] instructions to a large language model [LLM], which can then synthesise and understand the relationship between tables and suggest what a potential data schema could look like,” said Srivathsan.
She added that GenAI tools could reduce cloud migration time by about 30-40%. “As LLMs mature and more use cases and ready-made tools emerge, the time to migrate workloads to the public cloud will continue to decrease, and hopefully, the migration process will become more efficient,” she said.
In addition to cloud migration, GenAI could also help address skills shortages. For instance, using Amazon Kendra, organisations can synthesise their documents to help employees with older technical skills learn new technology concepts using prompts. Other common GenAI use cases include coding, content creation and customer engagement.
Hyperscalers like Amazon Web Services (AWS) and Google Cloud already offer model gardens and various AI platforms for organisations to build, train and run their own models, making it easier for organisations to tap the benefits of GenAI.
Read more about AI in APAC
- AI can be transformative in government, but its implementation has to be done in a way that drives positive outcomes while mitigating its downsides, according to the head of Australia’s Digital Transformation Agency.
- Culture Amp is building a generative AI capability that summarises employee survey responses, automating a process that typically takes HR admins up to hundreds of hours to complete.
- Firefighters from the Singapore Civil Defence Force will employ smartglasses equipped with computer vision and 5G capabilities to perform visual inspections of their equipment.
- The multidisciplinary nature of AI offers career opportunities not only in builder roles like engineering and data science, but also in AI ethics and applied AI.
Srivathsan said the cloud remains the ideal way to start with GenAI. Attempting to do it in-house due to proprietary datasets and concerns about security, data privacy and intellectual property infringement may limit scalability and flexibility. The industry-wide shortage of graphics processors could also pose challenges.
She also provided insights into how organisations are deploying GenAI models. They often begin with off-the-shelf models for one or two use cases to prove a business case before scaling up across the organisation. They are also fine-tuning models with proprietary data and performing inferencing in hyperscale environments to achieve scale and flexibility.
Over time, she expects organisations to host some models closer to their premises, potentially training them as inferencing occurs. However, she doesn’t think much inferencing will occur at the edge, except for mission-critical applications that demand ultra-low latency, such as autonomous driving and real-time decision-making on manufacturing floors.
Srivathsan stressed that organisations that implement cloud correctly by establishing the right security controls, data schemas and architecture decisions will be able to adopt GenAI more rapidly, creating a significant competitive advantage.
Selecting the right model will also be crucial to avoid excessive costs resulting from GenAI efforts. She advised organisations to identify the appropriate model for their specific needs to be cost-effective and efficient.
For organisations that deploy and fine-tune their own models, they should consider the data pipelines needed for launch and the datasets they plan to use.
“There is a lot of work that happens on the data side, and when it comes to MLOps [machine learning operations], you’d also want to start thinking about alerting the operations team if developers are touching the data or doing something funky with the models that they shouldn’t be doing,” said Srivathsan.