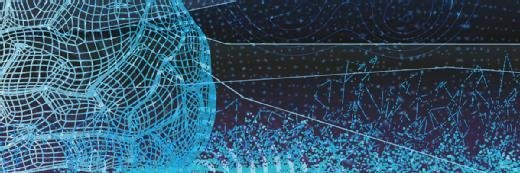
How to find winning artificial intelligence use cases
Choosing use cases for artificial intelligence requires organisations to take a hard look at business value, risk, data and other capabilities required to realise the technology’s full potential
A global study by Gartner last year showed that finding use cases for artificial intelligence (AI) and achieving returns on AI investments were the top two barriers for organisations in their AI adoption journey.
Security and integration with existing applications, the top two barriers revealed in previous studies, were no longer among the top barriers, underscoring the growing maturity of AI adoption across the globe.
But to sustain their successes in AI, organisations will need to work hard at identifying winning AI use cases. That means taking a hard look at the business value of AI as well as the capabilities required to realise the technology’s full potential.
Speaking at the Gartner Data & Analytics Summit 2022 in Sydney, Australia, Erick Brethenoux, distinguished vice-president analyst at Gartner, presented a methodology to suss out and prioritise potential AI use cases, starting with assessing the business value of AI versus the risks involved.
While businesses can proceed with AI initiatives with high business value and low risk, those that have low business value and high risk need not be ruled out as long as there’s a competitive differentiator that can benefit an organisation.
He said that while Gartner has worked with organisations on many AI initiatives to grow revenue, such as optimising prices, forecasting sales and generating demand, it has also come across projects aimed at cutting costs, optimising resources and improving product quality.
Just as important is the feasibility of an AI use case, not only from a risk perspective but also whether an organisation has the skills, technology, as well as data – which may not be readily available due to privacy laws – to support the project from inception to deployment and use. “From there, we can derive the use cases that are no-brainers, or at least the ones we believe could be implemented faster at a lower risk, and which ones we should be more cautious about,” said Brethenoux.
Read more about AI in APAC
- Bosch India is leveraging advanced analytics and artificial intelligence to detect manufacturing defects and plans to tap quantum computing for material simulation.
- MSD’s global lead for artificial intelligence discusses what it will take for the technology to realise its full potential in healthcare.
- InnerVision Engineering’s smart parking solution uses machine vision and other technologies to deliver a frictionless parking experience for drivers in Australia.
- South Korean internet giant Naver has built a global research network to advance and apply its artificial intelligence capabilities across its services.
Gartner’s methodology may vary according to an organisation’s maturity and competencies, but surprisingly, 72% of respondents told the research firm in the same study that they did not have problems with finding AI experts.
“One of the reasons is because they are not looking for unicorns – they are training their people and upskilling them through Coursera, Udemy and certificate programmes at local universities,” said Brethenoux.
“These people already know most of the AI use cases; they have a network within the enterprise; they can hit the ground running and are less likely to be poached by others,” he added, noting that it’s easier to train subject matter experts on data science than for data scientists to pick up subject matter expertise.
Brethenoux also touched on data quality in his presentation, noting that the quality of data trumps quantity any time, enabling organisations to get up to speed with AI implementations quickly.
“Nine months is usually the time it takes to start a project and implement it,” he said. “It seems long, but it’s actually short for AI projects. That means the scope has been done right and a proof of concept has been done in around nine weeks.”
How fast an organisation realises the value of their AI initiatives will also depend on the relationship between AI experts and software engineers.
“AI experts see the world differently – they like to see how their models change, drift and evolve over time,” said Brethenoux. “Software engineers are much more pragmatic, and testing matters a lot to them, but testing, to data scientists and AI experts, doesn’t mean the same thing.”
Testing models
Brethenoux said data scientists test models by validating them against data they had set aside to ensure their models achieve high accuracy. But they don’t do that for implementations and applications.
“And when a model drifts, they don’t test it every time to see if it’s still good,” he said. “But software engineers expect that, so the dialogue between them is very important, with a lot of time and money spent on these two teams.”
One of the biggest problems Brethenoux sees with organisations that are choosing and implementing AI use cases is the inability to nail down a particular key performance indicator. That includes not only identifying the KPI, but also knowing who is measuring it and where it is measured.
“One of the data scientists I work with quite a lot is the chief data scientist of Verizon Wireless,” he said. “When she gets a request from the business side, she sit downs with the team for three hours, bombarding them with questions like, ‘Why do you need a model? Where is it going to be run? Which application is going to use it?’
“If I give you that model today, how will you know it’s going to be successful? How are you measuring your existing use case? And where am I going to make a difference? By asking all those questions, she gets out of the room knowing how it’s going to be measured.”