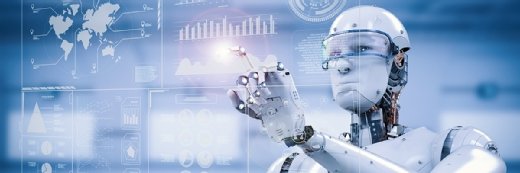
phonlamaiphoto - stock.adobe.com
How AI helps protect Japan’s road system from subsidence
Tokyo-based Kawasaki Geological Engineering is using Fujitsu’s Zinrai artificial intelligence system to develop a solution to erosion causing subsidence and sinkholes on Japan’s road network
Beneath Japan’s vast, complex (and often downright puzzling) road network runs an equally vast network of sewage and water pipes. But with much of this underground infrastructure laid in the post-war economic boom now old and decaying – as well as being subject to frequent earthquakes – subsidence and sinkholes on the surface are becoming a major source of disruption.
In 2015, according to Japan’s ministry of land, infrastructure, transport and tourism, road subsidence was identified at 3,300 locations around the country. In November 2016, to give just one example, a sinkhole that opened up in Fukuoka on the southern island of Kyushu blocked a major city centre intersection for days.
Water from deteriorating pipes flowing into subway tunnels beneath the road was held to be the cause of the Fukuoka street collapse, and it is possible, indeed likely, that loss of life was averted only because it happened at 5.15am.
But now new deep learning and artificial intelligence (AI) techniques are being applied to data generated by geophysical radar in the hope that the process will identify areas of erosion beneath road surfaces before it creates dangerous underground cavities, potentially averting life-threatening structural collapses.
So says Kawasaki Geological Engineering (KGE), a Tokyo-based engineering business that specialises in a diverse array of environmental surveying sciences, covering areas such as soil and groundwater contamination, sea-bed mapping, landslide and earthquake risk analysis, and structural deterioration.
KGE engineer Toshimune Imai says: “We needed to find a way to pinpoint cavities, because they cannot be seen on the surface of the road – so we have to find them before they collapse.”
To this end, KGE has developed its own ground-penetrating radar system, which is towed along behind a small minivan (pictured below) at a speed of 50-60kph. The 2m-wide array contains five antennae transmitting at a bandwidth of 50-1,000MHz, enabling them to penetrate 2-3m below the surface of the road to accurately pinpoint cavities caused by subsidence, groundwater leakage, and so on.
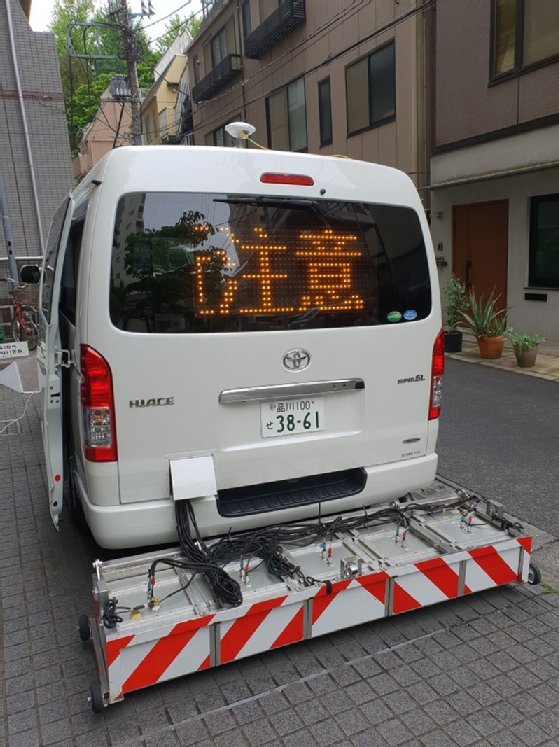
The data is collected on-board and then transferred back to the firm’s base – Imai hopes the advent of 5G will make this part of the job easier – but it is here that KGE hits something of a digital limit and reverts to analogue ways, as the data must then be printed out on sheets of A3 paper to be physically analysed by human technicians working in groups of three.
“If you look closely, you can see the responses that show there are cavities underground,” says Imai. “The slight bump depicts the fact that there is a cavity. But the shape is not limited to just that, and no matter how talented or well-trained they may be, the human engineers do get tired and there are times where they might overlook a cavity.
“Normally, multiple engineers would undertake the task of analysing the image results because there are times, if there is only one person analysing, that they may overlook something. This is why we use multiple engineers.”
With each 100m stretch of road generating one sheet of A3 paper, there was no way for KGE to get around the fact that it was using vast amounts of resources to do any meaningful analysis. KGE’s CEO, Toshihiko Sakagami, realised that things could not go on like this.
“That is why we started looking at AI,” says Sakagami. “Some of our engineers were knowledgeable about AI, so we had a fair idea that we could apply AI to detecting cavities in the same manner as people.
“The problem was that there was no commercial application, which meant we were unable to adopt the technology.”
With KGE’s first foray into AI best frankly described as a failure, Fujitsu’s traffic and road data service unit – with which it was already working on another project – suggested it try out the IT giant’s Zinrai AI platform.
Read more about AI
- The success of an online course in understanding artificial intelligence launched in Finland has led to a global roll-out.
- Nationwide invests in a startup developing AI that can work out how it should change the way it communicates with different people.
- Bosses don’t seem to understand that artificial intelligence needs good data management and a different understanding of end goals compared with traditional IT.
“The ultimate goal of a subsurface cavity identification AI is to never overlook a cavity,” says Imai. “But 100% accuracy is quite difficult in practice.
“On top of that, there are questions of how much accuracy can be improved and how far the false positive rate can be lowered, so ensuring that the quality of training data and the overall lack of training data have been issues in the development process.”
The lack of training data was probably the biggest challenge for KGE. As Imai puts it, had the engineers set out to train an AI to recognise pictures of cats and dogs, the job would have been far easier, simply because the internet is overrun with pictures of cats and dogs. Pictures of underground cavities – or, more specifically, radar images of them – are, by contrast, much rarer, and also not as cute.
There are also other things underground, such as chunks of stone or concrete, which create radar signals that humans might mistake for cavities. KGE therefore decided that abnormalities that humans mistook for cavities should also be included in the training data, effectively doubling the original amount of data and bringing down the false positive detection rate.
During test runs on this enhanced dataset, KGE found that the Zinrai AI was ultimately able to identify underground cavities with an accuracy of about 82%, compared with 80% for humans. This may not seem like a huge leap statistically, but at the same time it has reduced primary detection time for underground anomalies by 90%, accomplishing in seconds what used to take engineers hours. When the time taken to manually check the AI’s work is factored in, this is closer to a 50% time saving, still impressive by any measure.
Imai also notes that when human engineers are examining the printed data, they are often prone to looking into specific areas on the paper, potentially missing crucial information. “Equipping ourselves with AI adds objectivity to what we are analysing and trying to survey, which is another benefit,” he says.
Enhancing human competency
Sakagami emphasises that this does not mean KGE is cutting back on the number of engineers it employs. He takes the view that AI should “enhance the competency of humans”.
“Specialist engineers will always be needed,” he says. “Developing AI and developing engineers are two sides of the same coin for our business.”
Although the service has yet to be offered to a paying customer, Imai says the initial stages are to make the internal work as efficient as possible.
KGE is also working on a more compact, mobile version of the radar technology that can be installed on unadapted local government vehicles so that more data can be collected during day-to-day operations. It hopes this will result in a commercial product in 2021 or 2022.
“Our intent is to evolve this as a service to outside customers eventually,” says Imai.