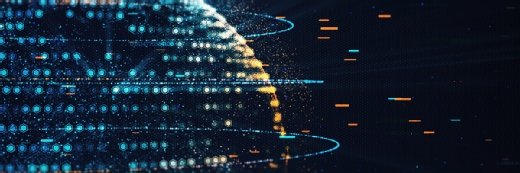
Getty Images/iStockphoto
Six ways to use LLMs operationally in the enterprise
Where are the business value, risk and deployment difficulties of LLMs? Here are some of Forrester’s top tips
Forrester predicts that operational support use cases for large language models (LLMs) will develop rapidly.
LLM integration with action components creates fully autonomous workplace assistants (AWAs) that become valued coworkers for support processes like finance and accounting, onboarding customers or field service implementation, and difficulty depends on the degree of input and output shaping.
Adoption timeframes are a function of four criteria: in production, near production, validated by an end-user company, and actively supported by the supplier.
Improve auditing efficiency
One example of operational use of LLMs is in finance and accounting to reduce external auditing fees. Every chief financial officer (CFO) wants to reduce external auditor billable hours. LLMs can answer auditor questions and reduce the hours and internal staff required to gather the information.
Trullion CEO Isaac Heller believes tangible areas such auditing as will be first up for LLMs. He cites a Trullion customer that took customer lease data from internal documents and used public data from an LLM to calculate complex interest rates and their lease liability. The combination allowed it to estimate the value of a leased asset for SEC reporting and financial disclosures.
In the long term, LLMs will be able to generate financial and planning scenarios and make a strategic difference. For instance, the LLM could be asked to estimate the effect of moving 10 full-time employees (FTE) from marketing to manufacturing on the fourth-quarter income statement.
Employee self-service
Another potential operational use for LLMs is in human resource (HR) policies that drive employee self-service. Today’s employee self-service chatbots depend on canned searches, which result in a high rate of unsuccessful queries. LLMs will replace these and answer questions like the number of PTO (paid time off) days remaining for parental leave.
Providers are planning to use multiple LLMs, and some have reduced hallucination rates to less than 3%. AWAs will coordinate application programming interfaces (APIs) and work patterns to core systems with employee data. For example, an LLM with narrow-model support for verification could ingest your HR policy.
A prompt with employee status and history from internal systems could ask for help to move a worker from part-time to full-time employment. Post-processing actions are left to the AWA to suggest a menu of actions orchestrating robotic process automation (RPA) bots or APIs to other systems.
Help for technicians
In field service, LLMs can guide various field repair use cases. LLMs can generate repair guidance for technicians at the appropriate skill level, an important talent that reduces training costs. For many repair scenarios, a technician can prompt an LLM with images or diagnostic data and ask for an answer appropriate for an expert technician or a novice.
Jim Fish, vice-president of Opus IVS, says: “Today, we use several LLMs in a multistep process. We feed the results in composite through another multistep process to refine the final answer.”
Opus has 21,000 field mechanics using this LLM in beta, providing a range of feedback. They have seen some hallucinations, but it is mostly positive input.
More focused customer communications
LLMs can also review prospect lists, raise customer experience (CX) with better customer context, or produce clearer and more personal customer communication. Prospect lists always contain unqualified leads. An LLM can eliminate them before a valuable sales effort launches.
For instance, a startup called Neto created an LLM-infused AWA called Ani, which a solar company uses to eliminate obvious dead ends. Wooded geographies, renters, or poor credit scores also take poor leads off the table. It’s a good example of the combined components needed for an AWA. Neto’s solution combines Twilio to handle communications, and Amelia, a conversational AI platform, to manage AI-driven process execution and logistics.
According to Akhil Tolani, Neto’s chief technology officer and co-founder, LLMs alone will never be safe enough for business. “Statistics will pick the next best word to use but can’t compute an overall confidence score. Inconsistency and hallucinations are therefore always possible,” he says.
Transcribing customer service calls
Another use case for LLMs is summarising customer conversions to eliminate post-call note-taking. For instance, customer service workers at an AT&T call centre recently stopped taking their own notes during customer calls. Instead, AI generated a transcript – which their managers could review – and suggested what the operators should tell customers.
Brett Weigl, senior vice-president and general manager for digital, AI and journey analytics at Genesys, a customer experience-as-a-service technology provider, says: “We see the potential for LLMs, and when it became practical to do so, we used them to summarise the calls between a customer and an agent. In most cases, the summary is about six lines of text, and not having the agent entering those notes provides the ROI [return on investment].”
Improve document clarity
LLMs can also be deployed to improve clarity, tone and comprehension for customer communications. For instance, insurance claims, complaints and customer onboarding can benefit from crisp writing with the right tone and reading level. Insurance death claims often have the same tone as a routine “fender bender”. Several suppliers are looking at tackling this.
Messagepoint’s Assisted Authoring supports configured prompts through a link to an LLM. Suggested rewrites or summarisation are returned. No customer data is sent to the LLM, and instructions guard against the LLM learning from and compromising corporate content and information.
Patrick Kehoe, executive vice-president of product management at Messagepoint, says: “The human-in-the-loop step is essential given where LLMs are today. We will test the approach to see if too much delay is introduced. European initiatives such as the UK’s Financial Conduct Authority Consumer Duty want more transparent and clear communication with customers. This can help.”
Risks and rewards
AWAs that leverage LLMs will have technical risks. When evaluating these, Forrester recommends that IT decision-makers avoid the pressure to use LLMs for everything and look to providers of narrow models for advanced help.
Enterprises risk pushing LLMs to places they don’t belong. A narrow model tailored to a common and high-volume document may suffice. For example, for invoice processing, why use an LLM for extracting information when a simpler extraction model is proven and requires less computer power and licence fees? Don’t hunt mice with an elephant gun. Forrester recommends that IT decision-makers use what they have or a simpler model if they can do so.
Read more about large language models
- Generative AI technology has already disrupted how enterprises work. While many companies are uneasy with the fast-evolving technology, that's expected to change.
- Healthcare professionals at Singapore’s National University Health System can now summarise patient case notes and predict patient healthcare journeys using a large language model trained by a supercomputer.
When looking at LLMs, there is no shortage of providers, but Forrester notes that strong support can come from suppliers that have been building custom models for years. Public models play an important role, but commercial models supported by AWAs with digital process automation (DPA) or RPA will tackle critical input and output shaping tasks.
According to Forrester’s July 2023 AI pulse survey, 16% of AI decision-makers whose enterprise has a generative AI (GenAI) strategy say their organisation is leveraging existing open source LLMs, while 42% are leveraging existing commercial foundational LLMs from suppliers. Amelia, for example, the conversational AI platform with AI-driven process execution, will host a public LLM for you as a private cloud solution, support required maintenance and training, and help with pre-processing.
Forrester recommends using existing AWA suppliers to experiment with DPA. RPA and enterprise application suppliers are integrating LLMs. For example, RPA suppliers want all workers to have a digital assistant, extending the task automation that established the market. LLMs may be the push they need.
This article is based on the Forrester report, “Operational processes will be the near-term LLM sweet spot”, by Craig Le Clair, a vice-president principal analyst at Forrester.