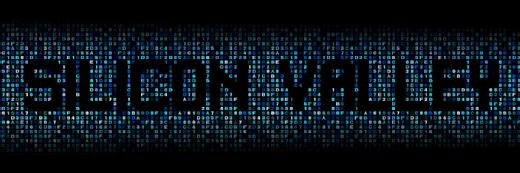
Stephen Finn - Fotolia
Silicon Valley startups aim to make big data capture and prep slicker
A group of California-based startup and early-stage data analytics and management companies are bidding to make big data, including sensor data, more tractable for analysis
Startup and early-stage data management and analytics companies in Silicon Valley continue to develop novel ways of tackling big data – with time-series data, data preparation and machine learning currently to the fore.
Computer Weekly was represented on a recent European IT press visit to data-focused companies in San Francisco and the Valley. As always, lineaments of the future of UK enterprise IT might be discerned in the fog of San Francisco and the eternal sunshine of Silicon Valley – save when, of course, it is being biblically flooded, as it has been in recent months.
InfluxData
San Francisco-based InfluxData, founded in 2013, takes the view that time-series data has mostly evaded even such modern technologies as NoSQL databases, and so requires a specific focus. The company describes this as “delivering a modern open source platform for metrics and events”. (A time series is academically defined as “a sequence of observations ordered in time”).
Paul Dix, the firm’s chief technology officer, said that although InfluxData has deliberately taken inspiration from NoSQL database MongoDB’s ease of use for developers, it is what it sees as the shortcomings of such databases – including Cassandra and CouchDB – that has given shape to a market opportunity.
InfluxData’s technology stack comprises a mix of open source and proprietary software – Telegraf (for the collection of sensor data), its own InfluxDB, Chronograf (for data analysis) and Kapacitor (for monitoring and taking action on data).
Evan Kaplan, chief executive officer at the firm, said there are three technology landscape changes in play that give context to the company’s offer. The first is a shift to microservices in application development; the second is an infrastructure change from mainframes to containers, via servers and virtual machines; and the third is the emergence of “the instrumented world” of the internet of things (IoT) – “the most significant trend long-term. Sensors are time series data”.
Kaplan also described the IoT as “ephemeral by nature”, adding: “It is expensive to evict data, and this is an ill-understood feature of this market.”
InfluxData has 250 paying customers, including BBC News, Barclays and SAP Hybris. A reference use case is at US clothing retailer Nordstrom, where it is used for DevOps monitoring. Lisa Gao, developer manager at Nordstrom, said: “We use InfluxData company-wide to gather operational metrics for the business. It is fast, requires minimal space, and has a rich retention policy capability.”
Crate.io
Crate.io is another database company focused on machine data. It has bases in San Francisco, Berlin and Dornbirn, Austria. It is targeting what it sees as a $1bn-plus machine data management market with an open source SQL database on NoSQL architecture.
Christian Lutz, chief executive officer and co-founder, and Andy Ellicott, vice-president for strategy and marketing, position the company’s technology as correlative to a second generation of big data, which mainly concerns machine data.
Ellicott said: “We often replace MongoDB and Cassandra. It is hard to make those databases work for you [with machine data].” He also seeks to differentiate the firm from InfluxData, which he says is “great for time series, but that’s it. It’s not good for geo-spatial data, for instance”.
Lutz said that although “Mongo is good for consistency and Couch for availability”, SQL is still the most widespread skillset among database developers. He added that while “you don’t get the full SQL technology set with us”, he felt Crate.io’s technology was still “more democratic”.
As always, there is the question of how robust the use of open source as a revenue model can be. “It is a double-edged sword,” said Lutz. “But no one who chose us would have done so if we had not been open source.” Crate.io has about 50 production customers at present.
GridGain
GridGain, based in Foster City, is another open source supplier that has a focus on in-memory database technology. It was founded in 2011 by Russian-born Nikita Ivanov and Dmitriy Setrakyan, and is a contributor to the Apache Ignite project, which it set up in 2014 with a code donation.
Abe Kleinfeld, chief executive officer at GridGain, is a Silicon Valley industry veteran, with previous CEO roles at nCircle and Eloquent. He described the company’s technology as a “fabric” between applications and data, which uses in-memory computing to speed up throughput and reduce latency in big data systems.
“Why now for in-memory?” he said. “In a word, cost, which has been dropping 30% every 12 months. We are the open source alternative to SAP Hana.”
Read more about learning from Silicon Valley technology developments
- Back to basics: What is Silicon Valley?
- Companies based in Silicon Valley discuss the issues created by big data technologies as they mature.
- We visit Silicon Valley to meet some of the emerging tech companies focusing on enterprise applications.
- California startup and early-stage data analytics companies are positing technologies that point beyond second-generation business intelligence, such as Qlik and Tableau.
One reference customer is Sperbank, a Russian bank that chose GridGain’s in-memory architecture ahead of SAP and Oracle, said Kleinfeld.
On the non-traditional database front, GridGain founder and CTO Ivanov said that at Apache Ignite, “we add transactions to Cassandra and speed it up – and our support for all major programming languages is important to our larger story”.
Ivanov said the Ignite community is also looking at artificial intelligence and machine learning, with developments expected some time this year.
Trifacta
San Francisco-based Trifacta, whose leadership team includes co-founder Joe Hellerstein – a chair in computer science at UC Berkeley – as chief strategy officer, is focused on data wrangling – sorting data out and getting it into shape for analysis. Chief executive officer Adam Wilson says the company’s founding principle is: “The people who know the data best should do the wrangling.”
Its customers include the Royal Bank of Scotland, Unicredit, Santander and the Luxembourg Stock Exchange in financial services; others include PepsiCo, Walmart, Target and LinkedIn. Bertrand Cariou, senior director for solutions and partner marketing, said 60-70% of Trifacta’s European customers are in banking and insurance.
The company featured in a press visit that included Computer Weekly last year. This year, Sachin Chawla, vice-president engineering, said the company’s roadmap included [data wrangling] task suggestion, for users of its software, based on machine learning, and more on governance, including with partners, such as WaterlineData.
In the same week as this year’s visit, the company announced at Google Next 2017, also in San Francisco, the launch of a collaboration with Google, dubbed Google Cloud Dataprep. This embeds Trifacta's interface and its so-called Photon Compute Framework, and natively integrates Google Cloud Dataflow for what it describes as “serverless, auto-scaling execution of data preparation recipes with record performance and optimal resource utilisation”. It enables analysts to explore and prepare diverse datasets within Google Cloud Platform for uses including analytics and machine learning.
Waterline Data
Waterline Data, founded by Alex Gorelik – who, like some of the Trifacta team, has a background at data integration pioneer Informatica – in 2013, tries to answer the question of how to get business value out of big data repositories. The company says its “catalogue” technology “discovers and raises trusted data above the waterline so you have the data you need to effectively run your organisation”.
It adds: “We automate the discovery, matching and tagging process and ensure the catalogue is always up to date by incrementally scanning the data itself and not just historical SQL logs.”
Chief operating officer Kaycee Lai said a typical Waterline Data customer will say: “We had a data lake, but no one was using it because they did not know what was in it.”
One reference customer is CreditSafe, an international company credit-checking service based in Cardiff, with offices in 14 countries and data from 100-plus countries.
Angus Gow, its chief technology and content officer, said Waterline’s software has been important in bringing a higher degree of automation to the management of CreditSafe’s data sources.
It started deploying the technology in November 2016 and plans to roll it out globally over the next two to three years. Evaluating company creditworthiness can be a locally inflected business, said Gow, who gave the example of Mexico, where salient factors can include how many company cars or how many windows a company’s offices have.
Profiling 450 million companies in the US using Oracle would have taken 20 days, said Gow, but using the Waterline cataloguing software reduced this to eight hours.
“If we had tried to build something similar ourselves, it would have taken 18 months to two years to do so,” he said.
ThoughtSpot
Scott Holden, vice-president of marketing at Palo Alto-based ThoughtSpot, said he is pleased that, after two years of active selling, the company this year made its debut on Gartner’s magic quadrant for BI and analytics.
Last year, Gartner made the controversial move of making its magic quadrant on business intelligence and analytics platforms about what it called “modern” BI. So, it was out with the old systems of record business intelligence systems, such as SAP Business Objects, IBM Cognos and Oracle Business Intelligence Enterprise Edition. Instead, pride of place was given to Qlik and Tableau, and the Qlik-and-Tableau-like software developed at the more comprehensive enterprise software suppliers, including SAP and IBM.
Holden described ThoughtSpot as part of a third wave of “postmodern” BI, which in its case is about being a “Google for numbers”, used by ordinary business users, not data scientists or, indeed, analysts; and marshalling machine learning to do that.
“Four out of our seven founders came from Google,” he said. “They are search people, not BI people.”
ThoughtSpot’s UK customers include financial derivatives dealer CMC Markets and car insurance firm Insure the Box, said Holden.
From the capture of machine data, through leveraging in-memory computing and wrangling data, to trying to make big data more user-friendly for analysis and taking action, this group of Silicon Valley-based companies are advancing offers at the forefront of modern, or indeed “postmodern” data management and analysis.