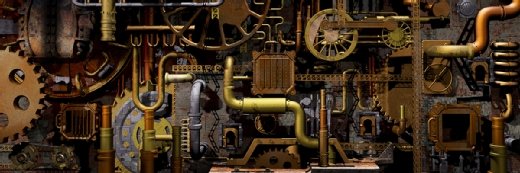
Kapley - stock.adobe.com
AI elevates predictive maintenance for Kone and ThyssenKrupp
Kone and ThyssenKrupp are using artificial intelligence to improve predictive maintenance of their lifts and escalators
Two hundred and sixteen centuries is a long time to wait for a broken lift. That figure is an estimate of the cumulative annual downtime of the 12 million elevators in the world which, if they are working, carry about a billion people every day.
Such is the impact of outages of elevators, escalators and travellators that two of the world’s leading manufacturers, Kone and ThyssenKrupp are looking to artificial intelligence and machine learning to predict failures before they happen. Their efforts hold lessons for the application of these technologies in predictive maintenance across a broad set of industries.
Predictive maintenance is nothing . Asset-intensive industries such have oil and gas have used statistical analytics tools to try to forecast outages and focus their maintenance efforts better for more than a decade.
But machine learning brings a level of accuracy and productivity to the approach, says Hyun-Shin Cho, ThyssenKrupp’s head of digital operations.
“The beauty of machine learning is it allows us to do predictive maintenance at scale on a large asset base,” says Cho. “Not only is it possible to come up with common failure pattern across hundreds of thousands of elevators, but by using anomaly detection algorithms, we can also develop failure patterns that are specific to each asset.
“Even if two elevators are the same model and make, they will have different usage patterns and subcomponents. You cannot apply a single set of rules to this vast heterogeneous environment – that is where machine learning is groundbreaking for us.”
Previous efforts in predictive maintenance have relied on identifying thresholds on a set of measures that would indicate a probability of failure across a broad set of assets.
In 2015, ThyssenKrupp launched a service based on data from internet of things (IoT) sensors, control system data, and data from the company’s broad environment of enterprise resource (ERP) and customer relationship management (CRM) systems, which include software from SAP and Oracle.
It has created a cloud-based data repository in partnership with Microsoft, based on the software firm’s Azure cloud platform.
“Instead of giving a computer system a set of instructions written in stone, machine learning allows us to take the data and have a system that can learn on its own,” says Cho. “We don’t say what failure patterns look like, we say here is the historic data and label where a system has failed.”
Read more about predictive maintenance
- What is Predictive maintenance?
- How Italy’s primary train operator, Trenitalia, combines the internet of things (IoT), analytics and in- computing in a project to make maintenance of its trains more efficient and effective.
- How National Grid uses cloud-based analytics from IBM SoftLayer to provide preventative maintenance.
ThyssenKrupp provides this service to about 120,000 elevators and other systems – some 10% of its asset base. “If you wanted to set up rules for each individual elevator, it would not be possible to do this with an expert [using statistical tools],” he adds.
ThyssenKrupp uses open source machine learning algorithms to build classification and regression models. It employs a combination of models across different data streams and classes of asset and assembles the output of these models to generate a combined result.
“Predictive models also get stale,” says Cho. “We need to retrain them constantly because the action we take while maintaining the assets will make them behave differently in the future.”
The aim is to get an engineer to site before the asset breaks down. Even if ThyssenKrupp’s predictive maintenance system – dubbed Max for its customers – fails to do that, clients often find an engineer is on the way when the make the call, says Cho. When the engineer arrives, the system has already done much of the diagnostic work that would otherwise have to begin when they get to the customer.
But to make a success of using IoT and machine learning to improve predictive maintenance, organisations have to break down some internal boundaries between enterprise IT and operational and service engineering, as well as other departments, says Cho.
“It is not technology driven,” he says. “Since day one, we included everyone. Field technicians were an important part – we wanted to change how they work, so they had to be at the centre. We had IT teams; we hired people with cloud expertise to bring the data together.
“You start small with the use case and it develops a gravity of its own and pulls everyone in – HR, legal, compliance, IT and operational teams. You need co-operation from everyone to make it a success.”
The outcome has been to create savings and efficiencies in ThyssenKrupp’s field service and maintenance operations, but also to offer higher service levels and lower downtime for customers buying into the service.
But it was not only developments in machine learning that made it possible, says Cho. The reduction of mobile data costs and developments in cloud technology were also factors, as was the acceptance of digital technologies by field engineers. “All this needed to come together to make it practical,” he says.
Kone and IBM
The confluence of these technologies has not escaped ThyssenKrupp’s competitors in the field. Kone, a fellow giant in elevator, escalator and travellator manufacturing and operation, has developed its own offer in partnership with IBM, based on its AI Watson IoT system (see case study below).
The ease of access to machine learning and AI in the cloud is helping to spread the reach and affordability of predictive maintenance.
PTC, a software company with a history in CAD/CAM technology and control systems, is now offering IoT, augmented reality and predictive maintenance to a much smaller set of businesses than global manufacturing or asset-intensive industries.
Kurt Bager, vice-president of IoT in Emea at PTC, says: “If you look at the IoT two years back, everyone knew they needed to connect everything – that was the hype. We saw many pilot projects of companies dragging in data. Now, this connectivity done, they ask: ‘why are we doing this?’
“Broadly, there are two reasons – cost reduction and to generate more revenue. The priority is cost. If you can do smarter maintenance or predictive maintenance, you can reduce cost. Nine out of 10 the business cases for IoT we see are around predictive maintenance.”
PTC applies open Apache Spark source machine learning algorithms extracting data from its cloud-based IoT platform. The technology’s easy access and low cost open it up to new users – those who do not necessary have large data science teams, says Martin Rand, PTC’s business development executive for analytics, Emea.
“You can get value out of the data without having to hire data science people or involve IT or R&D,” he says. “Machine learning enhances data discovery, aiding understanding of how equipment is being used or misused. If you find the patterns, you can also build predictive models.”
Wider pool of users
Neil Ward-Dutton, research director at MWD Advisors, says the application of AI and machine learning, together with cloud computing, has the potential to make predictive maintenance affordable to a wider pool of users. As the same time, new sources of data are constantly coming on-stream. For example, machine learning can be applied to recognition of infrared camera images.
“But it’s never as easy as it looks,” he says. “It comes down to economics. Any kind of investment in predictive maintenance is a cost and has to deliver a return. You find these decisions are bound up with bigger questions, such as the consideration of whether to outsource maintenance or do it yourself.”
Organisations also need to consider whether they have enough data from each asset or machine to build effective predictive models using machine learning, says Ward-Dutton.
“We see growth in this , but it has not stepped across the Rubicon. The tools are getting easier to use and are more widely available, but it is not the case that anyone can pick them up and make a go of it,” he says.
“You still need to make important choices in the kind of model you use, how you train them and how you clean the data. These are quite specialised skills.
“Of organisation that are using big plant or large assets, three-quarters are not using predictive maintenance in any serious way. There is still a lot opportunity in these industries before it spreads to other areas.”
Kone and ThyssenKrupp are showing that asset-intensive industries can use AI and machine learning technologies hosted in the cloud to start to build predictive models that help plan maintenance more effectively, potentially avoiding failures before they happen.
But challenges in developing and applying data models mean these techniques are likely to be applied where returns are greatest.
Case study: IBM’s Watson helps Kone avoid failures
In February 2017, Kone launched a partnership with IBM that saw the Finnish manufacturer of lifts, escalators and travellators take advantage of the computer firm’s artificial intelligence (AI) technologies to help predict when equipment might fail and therefore make maintenance more efficient.
With net sales of €8.9bn and 55,000 employees, Kone maintains 1.2 million pieces of equipment worldwide. They might be in hotels, airports, hospitals or residential buildings, all with different patterns of usage.
Customers are already seeing fewer failures and higher service levels, says Mikko Aro, Kone’s head of maintenance development. “In many cases, we have been able to detect failures in advance and prevent them happening,” he says. “In the old world, they would have happened.”
Kone has equipped assets using the service with IoT sensors that measure about 200 different parameters, picking up movement, temperature and forces within the machinery. It can then transmit the necessary data to its IoT cloud platform. It also collects data from control systems, which can generate error codes.
However, IBM Watson’s natural language processes and machine learning was also able to analyse information held in maintenance records and manuals going back tens of years, says Aro.
Bret Greenstein, global vice-president, IBM Watson internet of things, says that as well as natural language processing, AI can be applied to images, sound and vibration patterns.
With some components, such as rotating equipment, data scientists can start with generic models, and refine them using data specific to the machine, he says. As more data is included, models are refined to become more accurate for each asset.