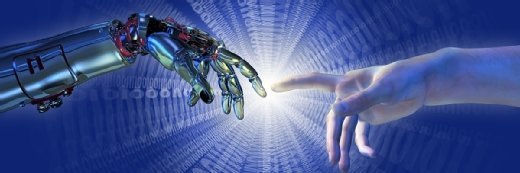
AlienCat - stock.adobe.com
Why new EU rules around artificial intelligence are vital to the development of the sector
Shawn Tan, CEO of global AI ecosystem builder Skymind, explains why the European Union’s new rules on artificial intelligence are a good thing
European Union (EU) lawmakers have introduced new rules that will shape how companies use artificial intelligence (AI). The rules are the first of their kind to introduce regulation to the sector, and the EU’s approach is unique in the world.
In the US, tech firms are largely left to themselves, while in China, AI innovation is often government-led and used regularly to monitor citizens without too much hindrance from regulators. The EU bloc, however, is taking an approach that aims to maximise the potential of AI while maintaining privacy laws.
There are new regulations around cases that are perceived as endangering people’s safety or fundamental rights, such as AI-enabled behaviour manipulation techniques. There are also prohibitions on how law enforcement can use biometric surveillance in public places (with broad exemptions). Some “high-risk” cases also face specific regulatory requirements, before and after entering the market.
Transparency requirements have also been introduced for certain AI use cases, such as chatbots and deep fakes, where EU lawmakers believe risk can be mitigated if users are made aware that they are interacting with something that is not human.
Companies that do not comply with these new rules face fines of up to 6% of their annual revenue – higher penalties than those that can be levied under the General Data Protection Regulation (GDPR).
Like many other firms in the AI sector, we are in favour of this type of legislation. For far too long, there have been too many cases where biased datasets have been used by companies to develop AI that discriminates against the society it is meant to serve. A good example was when Goldman and Apple partnered to launch a new credit card. The historical datasets used to run the automated approval process for the cards were biased and favoured male applicants over women, shutting out millions of prospective users.
These negative outcomes are a wake-up call for companies, and proof that they must seriously consider algorithm interpretability and testing. New, robust legislation puts a renewed sense of responsibility on those developing and implementing AI to be transparent and call out biases in datasets. Without legislation, companies have no incentive to put in the extra resources required to overcome such biases.
Reducing bias in AI
We believe that legislation can enforce ethics and help to reduce the disturbing amount of bias in AI – especially in the world of work. Some AI recruitment tools have been found to discriminate against women because they lean towards favouring employees similar to their existing workforce, who are men.
And it does not stop at recruitment. As ProPublica unveiled a few years ago, a criminal justice algorithm deployed in Broward County, Florida, falsely labelled African-American defendants as “high risk” at nearly twice the rate that it mislabeled defendants who were white.
Beyond the problematic issues of bias against women and minorities, there is also the need to develop collectively agreed legal frameworks around explainable AI. This describes humans being able to understand and articulate how an AI system made a decision and track outcomes back to the origin of the decision. Explainable AI is crucial in all industries, but particularly in healthcare, manufacturing and insurance.
An app might get it wrong when recommending a movie or song without many consequences. But when it comes to more serious applications, such as a suggested dental treatment or a rejected application for an insurance claim, it is crucial to have an objective system for developing more understanding around explainable AI. If there are no rules around tracing how an AI system came to a decision, it is difficult to pinpoint where accountability lies as usage becomes more ubiquitous.
The public is arguably growing more suspicious of an increasingly widespread application of biometric analysis and facial recognition tools without comprehensive legislation to regulate or define appropriate use. One example of a coordinated attempt to corral brewing collective discontent is Reclaim Your Face, a European initiative to ban biometric mass surveillance because of claims that it can lead to “unnecessary or disproportionate interference with people’s fundamental rights”.
When it comes to tackling these issues, legislation around enforcing ethics is one step. Another important step is increasing the diversity of the talent pool in AI so that a broader range of perspectives is factored into the sector’s development. The World Economic Forum has shown that about 78% of global professionals with AI skills are male – a gender gap triple the size of that in other industries.
Fortunately, progress is being made on this front.
Initiatives to counteract biases
A welcome number of companies are coming up with their own initiatives to counteract biases in their AI systems, especially around the area of career recruitment and using machine learning to automate CV approval processes.
In the past, traditional AI applications would be trained to stream resumés and if there were any biases in the datasets, then the model would learn them and discriminate against candidates. It could be something like a female-sounding name on a CV that the system is streaming. Subsequently, the system would not permit the hiring of that potential candidate as an engineer because of some implicit human bias against that name, and the model would therefore discard the CV.
However, there are standard ways to prevent these biased outcomes if the data scientist is proactive about it during the training phase. For example, giving an even worse score if the prediction is wrong for female candidates or simply removing data such as names, ages and dates, which shouldn’t influence hiring decisions. Although these countermeasures may come at the cost of making the AI less accurate on paper, when the system is deployed in a place that is serious about reducing bias, it will help move the needle in the right direction.
The fact that more innovations and businesses are becoming conscious of bias – and using different methods such as the abovementioned example to overcome discrimination – is a sign that we are moving in a more optimistic direction.
AI is going to play a much bigger part in our lives in the near future, but we need to do more to make sure that the outcomes are beneficial to the society we aim to serve. That can only happen if we continue to develop better use cases and prioritise diversity when creating AI systems.
This, along with meaningful regulations such as that imposed by the EU, will help to mitigate conscious and unconscious biases and deliver a better overall picture of the real-world issues we are trying to address.
Shawn Tan is CEO of global AI ecosystem builder Skymind