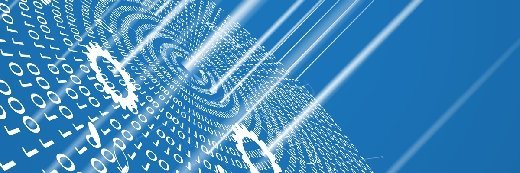
Fotolia
Vast Data launches into AI stratosphere with AgentEngine
Vast has built out into data management from its roots and will now offer customisable agentic AI agents that can tap into its existing storage, database and messaging capabilities
Vast Data has announced an agentic AI application environment – the Vast AgentEngine – for its data management stack, which ultimately rests on its Vast Data Store storage subsystem family.
The move marks a further foray out into the world of data management and applications, with special focus on AI and handling vector data that builds on existing planks in the company’s offer.
AgentEngine – which will be available in the second half of 2025 – allows customers to deploy and manage agentic AI agents. Vast plans to release a pre-configured agent monthly, but customers will be able to build them out to their own requirements. So, for example, they can create an agent, make MCP-compatible tools available to it – such as directory, S3 bucket, RAG pipeline, search, cataloguing and function tools – and assign a reasoning model to it, with AI frameworks and guardrails then able to be added.
All of this builds on the core elements of Vast’s offer. These are the Vast Data Store, which is PB-scale storage using high-density QLC flash and storage-class memory with “write shaping” to optimise performance and lifespan; Vast DataBase, with a range of options that include SQL, Kafka, Python and Parquet; and Vast DataEngine, a containerised, Python-based layer that brings scalable, event-driven computing on top of storage functionality. This can all exist in the context of what Vast calls the DataSpace, which can be deployed in the cloud or on-premise.
Vast co-founder Jeff Denworth described the resulting agents as providing very high level functions that will allow organisations to automate very mundane tasks.
“A good example is we’re working with a broadcast studio in the UK that’s asked us to create a video summarisation tool,” said Denworth. “So, if I want to watch all of my competitors’ channels and summarise them for producers, they don’t have to actually sit in front of the TVs to know what their competitors are doing.
“To do that, you need to be able to reason, you have to have large video language models, and then you need to contextually make decisions on what makes sense to summarise.”
Why release agents once a month? Surely there will be many different types of customer with various specialised needs? According to Denworth, some base functionality will exist in the agents released, but customers will be able to tailor them to their own use cases.
“We want to encourage adoption. The development environment is just a set of no code/low code tools,” said Denworth. “And the point is, it’s not that hard and there will be ISVs in the space, but there are a lot of customers that just want to roll their own and they don’t know where to get started. If we can develop something that’s 80% of the way there, or 70% of the way there, then that’s sufficient to get the conversation started.”
So, why is Vast reaching out this far into the application space? It started life as almost purely a storage provider but has gone way beyond that. In that, it’s not dissimilar to others that have become deeply involved in what might be considered data management. Agentic AI application building is a step further, however.
According to Denworth, storage was always just a starting point for a journey that would go high into the application stack.
“The reality is that we’ve been working with these since day one,” he said. “Our system is already heavily orchestrated and scheduled and was built with database structures since day one. The thing that we knew best was storage, and storage is the most important part of this because we wanted to build that new foundation that you could layer capability on top of.
“As we look at the ways that customers start to fall down as they go into AI, it typically relates to the data architecture. As an example, vector databases were never designed to be transactional, so if you have a business that is analysing and classifying data from the physical world in real time – it could be video, or whatever – if you don’t have a transactional vector database, then you can’t turn that into something a generative or agentic AI model can use.”
Read more about AI and storage
- Storage technology explained: AI and data storage. In this guide, we examine the data storage needs of artificial intelligence, the demands it places on data storage, the suitability of cloud and object storage for AI, and key AI storage products.
- Cloud storage for AI: Options, pros and cons. We look at cloud vs on-premise for AI workloads, why cloud is sometimes best, and the technologies speeding AI in the cloud such as parallelism and GPUDirect.