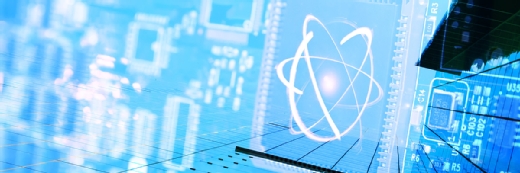
zapp2photo - stock.adobe.com
New machine learning algorithm to detect quantum errors
University of Sydney and quantum control startup Q-CTRL have developed a new way to reduce quantum computing errors using custom machine learning algorithms
Researchers at the University of Sydney and quantum control startup Q-CTRL have developed a way to identify sources of error in quantum computers through machine learning, providing hardware developers the ability to pinpoint performance degradation with unprecedented accuracy.
A joint scientific paper detailing the research, Quantum oscillator noise spectroscopy via displaced Cat states, was published in the Physical Review Letters, a physical science research journal and flagship publication of the American Physical Society.
Focused on reducing errors caused by environmental “noise” – the Achilles’ heel of quantum computing – the University of Sydney team developed a technique to detect the tiniest deviations from the precise conditions needed to execute quantum algorithms using trapped ion and superconducting quantum computing hardware. These are the core technologies used by industrial quantum computing efforts at IBM, Google, Honeywell and others.
To pinpoint the source of the measured deviations, Q-CTRL scientists developed a new way to process the measurement results using custom machine learning algorithms.
In combination with Q-CTRL’s existing quantum control techniques, the researchers were also able to minimise the impact of background interference in the process. This allowed easy discrimination between “real” noise sources that could be fixed and phantom artefacts of the measurements themselves.
“Combining cutting-edge experimental techniques with machine learning has demonstrated huge advantages in the development of quantum computers,” said Cornelius Hempel of ETH Zurich who conducted the research while at the University of Sydney.
“The Q-CTRL team was able to rapidly develop a professionally engineered machine learning solution that allowed us to make sense of our data and provide a new way to ‘see’ the problems in the hardware and address them,” he added.
Q-CTRL CEO and University of Sydney professor Michael Biercuk said the ability to identify and suppress sources of performance degradation in quantum hardware was critical to both basic research and industrial efforts building quantum sensors and quantum computers.
“Quantum control, augmented by machine learning, has shown a pathway to make these systems practically useful and dramatically accelerate R&D timelines,” he said. “The published results in a prestigious, peer-reviewed journal validate the benefit of ongoing cooperation between foundational scientific research in a university laboratory and deep-tech startups.
Q-CTRL, a spin-off from the University of Sydney’s quantum science group, is also partnering with Transport for NSW to look at how quantum computing could create and manage a more resilient transport network.
Future applications of the technology could include mapping all transport modes and crowd movements simultaneously in real time and automatically updating the schedule to solve disruption issues.
Australia is seen as one of the forerunners in quantum computing. In 2018, scientists from the University of Melbourne simulated the power of quantum computing on supercomputers to crack a mathematical problem that would have required the memory capacity of more than a billion laptops to solve.
The breakthrough was an important step to help researchers get “quantum-ready”, according to Lloyd Hollenberg, deputy director at the university’s Centre for Quantum Computation and Communication Technology.
“The capability to simulate quantum algorithms at this level is critical for learning how a quantum computer in the future will physically operate, how the software can work and what sort of problems it can solve,” he said.
Read more about quantum computing
- Industry experts predict it will take 10 years for quantum computing to become a reality, but Microsoft believes it has the edge to get there in five.
- Volkswagen Group recently demonstrated how it was working with D-Wave to research uses for quantum computing.
- Atos has made a quantum computing simulator available for sale so that organisations can test out the algorithms of the future.
- A top European CIO is urging the security community to prepare for quantum computing to ensure their encryption processes are ready in time.