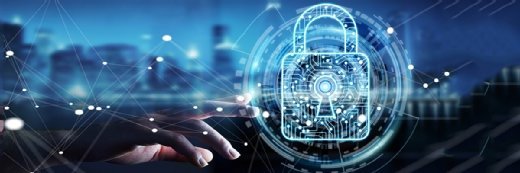
sdecoret - stock.adobe.com
Microsoft Build 2020: Focus on protecting data for AI development
Microsoft Build is always a vital event for developers across the IT industry. This year, Microsoft has expanded its AI emphasis
Microsoft Build took on a different feel this year because of its digital format as a result of the Covid-19 crisis. But the show was not short of news, especially in artificial intelligence (AI), privacy and responsible machine learning, which took centre stage.
Much of the existing narrative about responsible AI focuses on high-level areas such as ethics, policy and establishing principles for the technology. These are important, but often too abstract to carry any real-world relevance or provide operational guidelines for developers.
By contrast, Build saw a much deeper focus on the technical tools and practices to help AI practitioners build and deploy machine learning responsibly from the outset. Moves in this area form part of a wider push by Microsoft into responsible AI this year and, in particular, the tools to enable effective governance of machine learning applications and processes.
Let’s take a closer look at some of the key announcements and the tools Microsoft has developed for responsible AI. They have vital implications for businesses and the industry over the coming 12 months.
Responsible AI is a combination of principles, practices and tools that enable businesses to deploy AI technologies in their organisations in an ethical, transparent, secure and accountable way.
The area has been getting a lot of attention recently as more decision-makers consider introducing data and AI solutions in mission-critical and regulated areas such as finance, security, transportation and healthcare. Also, concerns are mounting about the ethical use of AI, the risks inherent in biased data and a lack of interpretability in the technology, as well as the potential for malicious activity, such as adversarial attacks.
For these reasons, the governance of machine learning models has become a top priority for enterprises investing in such systems. A survey of senior IT decision-makers in 2019 by my firm, CCS Insight, indicated that the two most important requirements when investing in AI and machine learning technology were the level of transparency of how systems work and are trained, and the ability of AI systems to ensure data security and privacy. These two requirements were cited by almost 50% of respondents.
One of the major areas on show at Build this year was Microsoft’s expanding portfolio of tools available in open source, Azure and soon natively integrated into Azure Machine Learning, that help data scientists, machine learning engineers and developers get hands-on experience of responsible AI.
The global company is focused on building trust and transparency into the entire lifecycle of machine learning, from data acquisition to modelling and deployment. Its tools focus on three principal areas – protect, control and understand – which saw several major announcements.
This area addresses scenarios in machine learning that involve sensitive information or privacy requirements, such as using personal health or census data. The data security, privacy and compliance capabilities of the Azure cloud are fundamental to Microsoft’s efforts, along with Azure Machine Learning, its platform for the operationalisation of the technology, from training to deployment and monitoring.
Read more about practical AI
- Microsoft AI Builder empowers non-programmers to incorporate AI as they build applications using low-code/no-code platforms.
- A new OpenAI partnership with Microsoft aims to provide OpenAI with an influx of capital to boost its AGI research and give Microsoft new supercomputing Azure AI capabilities.
One of the notable moves at Build focused on this area, and specifically on differential privacy. Differential privacy is a class of algorithms that facilitate computing and statistical analysis of sensitive, personal data while ensuring that the privacy of individuals is not compromised. Microsoft unveiled WhiteNoise, a library of open-source algorithms that enable machine learning on private, sensitive data.
As one of the strongest guarantees of privacy available, differential privacy algorithms are being adopted in several areas today. The US Census Bureau uses them to analyse demographic information and the likes of Apple, Google and Microsoft employ the technology to analyse user behaviour in their operating systems.
Last year, Microsoft partnered with Harvard University’s Institute for Quantitative Social Sciences to develop an open source platform to share private data with differential privacy to bring more researchers into the field. However, widespread adoption in enterprises is minimal, but with the release of WhiteNoise, Microsoft is aiming for more organisations to begin using the algorithms for machine learning on sensitive data.
Another major announcement was the unveiling of efforts to support confidential machine learning, which is coming to customers later this year. It enables the building of models in a secure environment where the data is confidential and cannot be seen or accessed by anyone, including the data science team. All machine learning assets, including the inputs, models and derivates, are kept confidential.
The capability adds to Microsoft’s approach to building models over encrypted data following its release into open source in 2018 of Simple Encrypted Arithmetic Library (SEAL), a set of encrypted libraries that allow computations to be performed directly on encrypted data using homomorphic encryption.
Nicholas McQuire is a senior vice-president and head of enterprise and AI research at CCS Insight.