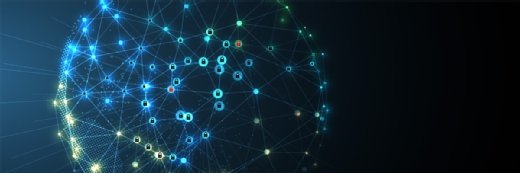
Perfect storm for data science in security
After nearly 20 years of research, the perfect storm of computing technologies is finally enabling data science to realise its potential to make valuable contributions to improving cyber security capabilities
As the focus in cyber security is shifting from threat prevention alone to detection and response, data science is playing an increasingly important role, according to Joshua Neil, principal data scientist lead for Windows Defender Advanced Threat Protection at Microsoft.
“I have watched the evolution of data-driven methods applied to cyber security from its early days, and I am excited to be part of that revolution,” he told Computer Weekly, adding that this approach is gaining momentum and efficacy as all the necessary underlying technologies become available.
In the past few years, Neil said there has been rapid progress from heuristic approaches using Boolean logic to encode rigid rule sets to match well-known attack behaviour against threat data.
“But in those early days we were being defeated – because it was easy to move around these heuristics-based defences – but data science has introduced a more general approach that is much more difficult to avoid or innovate around the defences, because we are asking more general and behavioural questions.”
Data driven approaches to cyber security have come a long way, said Neil, since he started working in the field as a statistician at the US Los Alamos National Laboratory, where he led an investigation into using data-driven approaches in cyber security, focusing on lateral movement of adversaries inside targeted enterprises.
This type of activity typically came to light only after attacks had taken place through intensive manual effort and expensive forensic investigations.
“In the early 2000s, we realised that first and foremost, we needed visibility into the enterprise, but only now is the industry making available the tools and technologies that enable the collection of the high quality data required to find malicious activity in automated ways,” said Neil.
Endpoint detection and response systems
Now, it’s finally possible to see into networks at high-resolution and at large scale, as well as capture the data using endpoint detection and response (EDR) systems, he said.
“That has given us the visibility and data we needed, and now we also have the cloud infrastructure needed to analyse that data. All these enabling technologies had to become available in parallel to enable us to be effective [with data science] in ways that were not possible in the early 2000s.”
The intervening years, said Neil, were difficult for those who had realised what data science could achieve if applied to cyber security, but who had no means of doing so yet.
“It took a lot of patience for those of us who had seen where we needed to get to, to wait for that technology to come along and mature enough, but now it is so exciting,” he said.
Read more about data science, security and privacy
- Security teams increasingly use large data sets from their networks to find hidden threats. Why companies should embark on their own data science and machine learning initiatives.
- The UK’s information commissioner remains in the top three data leaders after claiming top spot in 2018 in the DataIQ 100 list of most influential data leaders, but GDPR is not the only focus in the data science industry.
- In 2014, City University London introduced its first post-graduate courses in data science and cyber security, which will help businesses fill skills gaps as they try to get more out of the high volumes of data they have and protect its value against cyber threats.
Joining Microsoft 18 months ago, said Neil, was an important step in his data science career. “Finally, after 20 years I am at a company with the scale, enterprise visibility and cloud computing that I need to do my job and have the impact that I knew would be possible [with the right tools].”
As a result, Neil said there is much more recognition among enterprises of the potential of data-driven approaches to security, but this is not equally well understood by all.
“This approach is best understood by high risk industries, such as the financial services industry, where some of the largest firms have even started setting up their own cyber data science teams that are collecting and analysing the data to help improve cyber protections.”
However, in many cases, Neil said these organisations realise that this is a challenging and expensive thing to undertake on their own or they are not realising the full potential of data science for cyber security because their data scientists often new to cyber security and tend to work in isolation.
Applied correctly, he said, data science is helping to improve cyber security capabilities both pre- and post-breach.
“In the pre-breach context, data science is making a huge contribution in enabling organisations to identify malicious code by using supervised machine learning technology to extract behavioural features from suspicious executables run in isolated environments.”
This approach means organisations are no longer relying on signature-based malware detection, making it much more difficult for malware authors to evade detection.
Anomaly detection
Post-breach, anomaly detection is among the most successful applications of data science, said Neil. “Once attackers are inside the enterprise, they look like users. They are using valid credentials to access systems and data, and they are stealing that data using built in system tools, making it difficult to detect.”
Anomaly detection, he said, uses self-learning models designed by data scientists to understand “normal” behaviour inside the enterprise.
“This can be very high-resolution. Every user’s credential behaviour is modelled and the behaviour between every communicating computer on a network is modelled, creating hundreds of millions of models for any given enterprise to identify anomalous – and potentially malicious – activity.
“Supervised machine learning pre-breach and anomaly detection or statistical methods post-breach are the two big areas where I think we have made the best contributions in terms of detection,” said Neil.
Automated methods
Another key contribution by data science is in describing the extent of an attack as well as possible through automated methods. “Detection and response go hand in hand, and so the more we can detail the extent of an attack in terms of detection, the more we can accelerate the response.”
Data scientists are also working in the field of automated response, but Neil said in this regard, it is “still early days” and automated response remains highly dependent on detection capability.
“You need to be very sure of your detection before you start shutting machines down because a False positive here is quite expensive for the enterprise, so this is a real challenge.
“However, progress is being made, and Microsoft has some of these automated response systems deployed. But we are very careful about this. Automated response is a very long-term goal. Regardless of the hype, it is going to take us years to realise this fully.”
That said, Neil believes a lot of the manual, human-driven cyber attacks by teams of well-funded attackers will start to be replaced. “I think we are going to start seeing attackers using automated decision making.”
This in turn will create the opportunity for defenders to write their own attack bots that can be used to fine-tune their automated defences. “We can play this game of attack versus defence before the adversary does.”
Eventually, Neil believes artificial intelligence (AI) bots will be used in both defence and offence without a lot of human involvement. “This could be a blessing or a curse for defence, but one thing for certain is that the state of things is changing and it’s changing very fast,” he said.