Access your Pro+ Content below.
Royal Holloway: A novel approach to clustering malware behaviour to improve malware detection
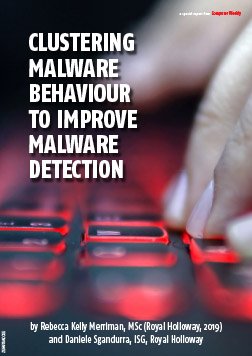

Sponsored by TechTarget ComputerWeekly.com
This article is based on a study to evaluate the accuracy of clustering-based malware detection to see whether it improves malware detection. Clustering malware behaviour can be very useful, but it is unknown how accurate clustering algorithms are when dealing with malware. In this article I describe an attempt to measure the accuracy of these algorithms. My results show that the accuracy of clustering-based malware detection is highly subjective.
Table Of Contents
- Due to huge amounts of data being available now (so-called big data) it is not practical to use traditional techniques or humans to analyse information. To this end, the rise in computational capacity and tools available to process and analyse data in real time have allowed us to use machine learning techniques to perform these tasks automatically.
- Machine learning and artificial intelligence will not only make cyber attacks more powerful but will also make cyber defence just as powerful.
- Due to the rise of the digital age, malware attacks are on the increase and, therefore, we need to find novel ways to detect and prevent these attacks from occurring in the future.
- To perform any machine learning algorithm, data needs to be processed to remove all irrelevant and redundant elements and to produce it in a form ready for input into the algorithm.
- The project: I used a dataset and performed dynamic analysis on three malware types. The distinguishing factor was the behaviour of the malware as each malware performed different actions.
- The accuracy of clustering-based malware detection is highly subjective as it depends on many factors including the type of machine learning algorithm, the selected features, the feature selection methods, the model construction methods and evaluation metrics.