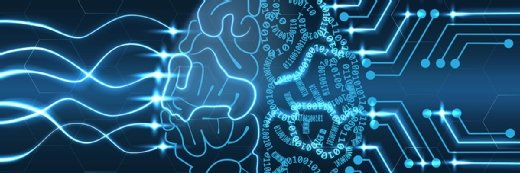
Laurent - stock.adobe.com
VMware and Nvidia say AI is maturing
This week's VMworld also included some announcements about artificial intelligence that should have repercussions for the channel
VMware and Nvidia have announced a pact at VMworld to make artificial intelligence (AI) a mass market.
AI is entering a phase of maturity where its early adopters have discovered most of the glitches and devised a way to work around them.
So virtual pioneer VMware has collaborated with graphics processing giant Nvidia (now part of Mellanox) to create a standard baseline platform on which all AI projects can be launched.
Until now, the installation of AI has been far too complicated to attempt for any company that doesn’t have global reach and the IT budgets to match. Companies need huge amounts of time and money to create the computing and network infrastructure on which to run all the software, host the data and process all the information, said Krish Prasad, VMware’s cloud business SVP and general manager.
This created a massive barrier to entry for most businesses. As a result, only the elite traders could afford to create AI-assisted recommending engines that upsell to customers with the words: “If you liked X, you may also like Y.”
It is these automated inventions that gave YouTube, Amazon and all the top online retailers the website “stickiness” that has enabled them to dominate markets. The neural networks behind these pioneering examples of AI have been unaffordable to most because there are so many configuration problems that need solving before they can be run in the cloud.
However, the process has been repeated often enough now for VMware and Nvidia to be able to create a common technology configuration onto which developers can run their AI systems. This brings down the price and timescale of developing AI considerably, said Manuvir Das, Nvidia’s head of enterprise computing.
Now its graphics processing units (GPUs) can be sliced and diced and mixed and matched to jobs running on the infrastructure, which means the computing assets are more fluid. That, he said, creates an internal market on the private cloud that has greater liquidity – which makes everything cheaper.
The upshot for the channel, said Das, is that the same level of “stickiness” can be installed on the websites of all kinds of smaller rivals. The combination of neural networks, comparative logic and recommendations could be used in a wide variety of sectors.
AI has matured and is ready to go mass market, which is great news for the channel, said Das. There will be endless possibilities and this partnership will shorten the project lifecycle, so you will get paid quicker, he added.
AI might have matured, but it needs to tidy its data before it starts its journey, said Louise Wright, head of data science at the National Physical Laboratory (NPL), the UK government’s measurement agency.
There are three stages of AI that a robot must go through. At birth, it has to be weaned on data. Like a human baby, it somehow amasses all this information and processes it and begins to make sense of the patterns that emerge. When it has absorbed enough information, it is trained (schooled) using the neural networks that enable machines to learn. Finally, it reaches a sufficient level of cognitive competence to be allowed to start making commercial judgements.
The last two phases of this three-part journey have been paved over by Nvidia and VMware, according to their recent announcement.
However, the data phase is crucial, said Wright. If you feed your fledgling automaton a diet of bad data, it will end badly.
Wright and the NPL are working to address this, offering recommendations to help UK industry avoid waste. But the problem with data is that it’s not standardised enough.
You can pick and choose the job, but nothing will ever be achieved, in whatever you do, without the right data quality, said Wright. That is why data professionals spend ages at the start of a project “washing their data” to get the rubbish out and trying to get all the records into the same format.
“Quality control is vital. Algorithms will crash without standards,” said Wright.
You all need to be speaking the same language about the same thing in the same way, he said – otherwise, the supply chain will be knackered.
Automated data cleaning can give you bias. On the other hand, you can end up with a rich set of data, but not the vital ingredients, which can mean the wrong data is being fed in. As an industry, we are still at the stage of defining vocal definitions, said Wright.
So there is an opportunity for the channel here, too. The upshot is that AI is about to become a mass market as it become more affordable, and there is plenty of sanity checking to be done.